Exploring Hidden Semantics in Neural Networks with Symbolic Regression
Yuanzhen Luo, Qiang Lu (luqiang@cup.edu.cn), Xilei Hu, Jake Luo and Zhiguang Wang.
Received: 24 March 2022 / Accepted: 14 April 2022 GECCO
Links
- Code: https://github.com/KGAE-CUP/SRNet-GECCO
- Paper: Exploring Hidden Semantics in Neural Networks with Symbolic Regression
- Appendix: Supplementary Material
Abstract
Many recent studies focus on developing mechanisms to explain the black-box behaviors of neural networks (NNs). However, little work has been done to extract the potential hidden semantics (mathematical representation) of a neural network. A succinct and explicit mathematical representation of a NN model could improve the understanding and interpretation of its behaviors. To address this need, we propose a novel symbolic regression method for neural works (called SRNet) to discover the mathematical expressions of a NN. SRNet creates a Cartesian genetic programming (NNCGP) to represent the hidden semantics of a single layer in a NN. It then leverages a multi-chromosome NNCGP to represent hidden semantics of all layers of the NN. The method uses a (1+$\lambda$) evolutionary strategy (called MNNCGP-ES) to extract the final mathematical expressions of all layers in the NN. Experiments on 12 symbolic regression benchmarks and 5 classification benchmarks show that SRNet not only can reveal the complex relationships between each layer of a NN but also can extract the mathematical representation of the whole NN. Compared with LIME and MAPLE, SRNet has higher interpolation accuracy and trends to approximate the real model on the practical dataset
SRNet Model
We present hidden semantics h of each layer in a NN as:
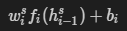
where f is represented by a CGP model, and w and b are a weight vector, as shown in figure:
For more details about our model and methods, please see our paper.
Experiments
We test our model at 12 regression tasks (SR Benchmarks and physical laws) and 6 classification tasks (PMLB), as shown in figure:
All the experiments code at here. Here we show our experimental figures in our paper:
Convergence
The convergence curves for all dataset:
Semantics (Mathematical Expressions)
Combining these expressions, we can obtain the overall expressions for all NNs:
Comparison
We compare SRNet to LIME and MAPLE on both regression and classification tasks.
Regression
Classifiation
Decision boundary:
Accuracy:
Cite
Cite this page:
Cite this paper:
1
2
3
4
5
6
7@inproceedings{Yuanzhen2022s,
title={Exploring Hidden Semantics in Neural Networks with Symbolic Regression},
author={Yuanzhen, Luo and Qiang, Lu and Xilei, Hu and Jake, Luo and Zhiguang, Wang},
booktitle={Proceedings of the Genetic and Evolutionary Computation Conference},
pages={xxx--xxx},
year={2022}
}
All articles in this blog adopt CC BY-SA 4.0 agreement ,please indicate the source for reprint!